Alumni, ISEF, Science Talent Search
Conversations with Maya: Lester Mackey
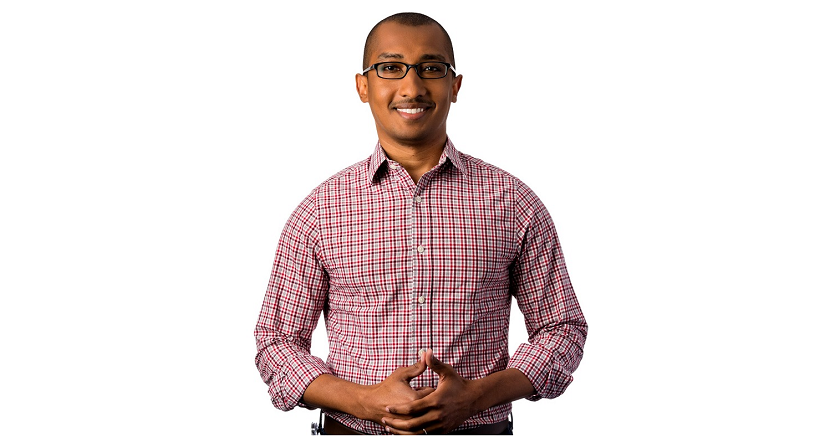
Maya Ajmera, President & CEO of Society for Science & the Public and Publisher of Science News, sat down to chat with Lester Mackey, a statistical machine learning researcher at Microsoft Research Labs New England. Mackey is an alumnus of the Science Talent Search (STS) and the International Science and Engineering Fair (ISEF), both science competitions of the Society. We are thrilled to share an edited summary of the conversation.
You’re an STS 2003 and ISEF 2003 alum. How did the competitions affect your life?
When I was a college freshman at Princeton, an Intel researcher reached out to me and asked if I wanted to intern in their Strategic CAD Labs. The lab was made up entirely of Ph.D.s and hadn’t taken on interns before. The researcher knew of me because of my participation in ISEF and STS, and if it hadn’t been for my science fair participation, I wouldn’t have had the opportunity to work there.
Long story short, I loved the experience. I liked the freedom that I had, the opportunity that I had to productively deploy creativity at every turn. And I came away from that experience determined to get a Ph.D. What’s more, my Intel mentor knew Maria Klawe, who was dean of Princeton University’s School of Engineering and Applied Science at the time, and recommended that she recruit me for a research project. That kicked off my research career.
Do you have any memorable experiences from the competitions to share?
My ISEF project was awarded an Operation Cherry Blossom Award, which included an all-expense-paid trip to Japan. This took me on an incredible adventure: We went to Tokyo and Yokohama and the ancient capitals of Nara and Kyoto. Part of the trip involved meeting a Japanese princess.
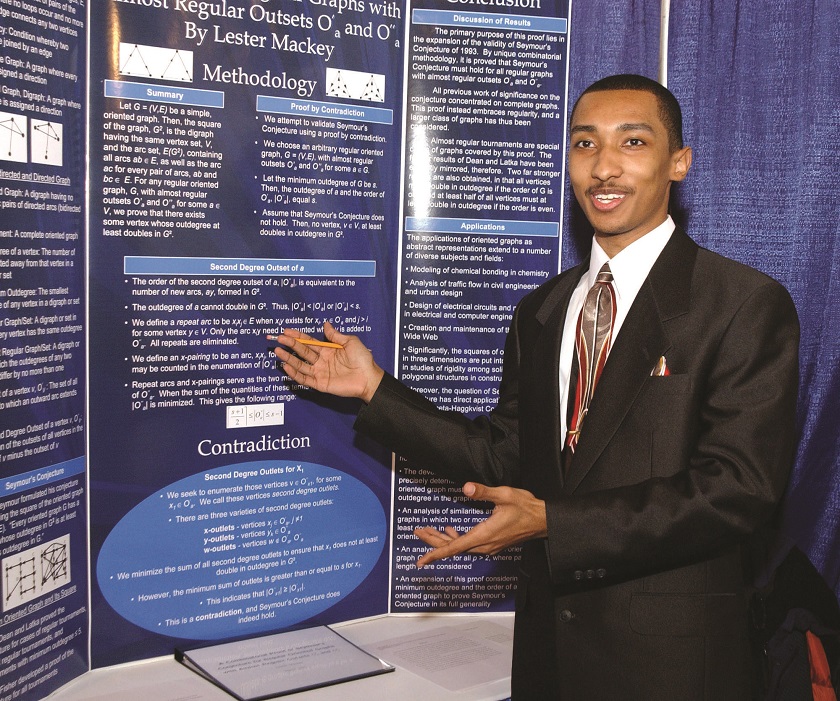
You taught at Stanford before moving to Microsoft. Can you describe how being at Microsoft has been different than a purely academic environment and how that’s helped you in your career?
In many ways my lab, Microsoft Research Labs New England (MSR), is very much like a university. The researchers here work on whatever they want. We publish everything. We’re evaluated based on our contributions to our academic communities and to the world.
The main difference is in the extra degree of freedom that we have at MSR. If you want to spend 100 percent of your time doing research, you can do that. If you want to teach courses at neighboring universities, you can do that too. You have the freedom to choose how you want to spend your day, and I think that freedom is very valuable.
I’ve also noticed that the researchers here tend to be very hands-on with their projects and very collaborative. I find myself working not just with my students, interns and postdocs, but also with my talented and experienced labmates. That’s been a big positive for me. Our lab is somewhat unique in that it was created as a research lab for both computer scientists and social scientists. Some of my colleagues are scholars in economics, communication or anthropology. The lab fits on a single floor, and that proximity breeds collaboration. I find myself working on problems that I hadn’t even considered before coming to MSR.
How do you recommend students start studying and getting involved in machine learning?
I tell all students to try a data science competition. My first encounter with machine learning was through a competition that Netflix ran when I was a senior in college. Netflix wanted to improve its movie recommendation system, so they released a dataset of 100 million ratings that users had given to various movies. Competitors were challenged to predict how the users would rate other movies in the future. My philosophy is that these public competitions provide a great sandbox for people who are just starting out in machine learning because you get to work with real data on a real problem that someone really cares about. By the end, you’ll understand both the methods and the problem, and you’ll have fun doing it.
There’s been a lot of talk about artificial intelligence and its influence on humankind. Why do you think students or the public should care about AI?
I think we have to be aware of these technologies so that we can hold them accountable to our standards of fairness and safety. AI is becoming much more pervasive, and it’s increasingly being incorporated into technologies that impact our everyday lives: self-driving cars, résumé-screening tools and algorithmic risk-assessment tools that inform bail-release and criminal-sentencing decisions.
I also think that AI holds the potential to help us address some of our biggest challenges like poverty, food scarcity and climate change. What I love most about my field is that these tools have the potential to do real good. I think that’s something that will excite many students and the public more generally.
Using AI to solve issues like poverty is interesting to think about. I would love to hear more about how this technology can be employed to solve these types of problems.
Take the example of GiveDirectly, a nonprofit that gives unconditional cash transfers to the poorest people in the poorest communities. They’re finding that this leads to sustained increases in assets. However, the on-the-ground process the organization goes through to identify transfer candidates is quite laborious and expensive.
So they’ve been working with experts in machine learning, statistics and data science to automate more of that process. Early work transforms satellite images into predicted poverty heat maps to guide the search of field-workers, and I think we’ve just scratched the surface of what is possible.
What do you feel are the most interesting problems that could be addressed within your field of research?
I’d love to see the field direct more of its attention and resources to social problems like poverty, hunger and homelessness. There are many open questions in this space. What specific problems could actually benefit from machine learning intervention? How can machine learners work with experts and policy makers to actually affect meaningful change? How do we incentivize our talented students and professional machine learners to work on these problems?
A second, different sort of challenge is responsible deployment. We see that AI is being used already to inform important decisions in society, such as screening résumés or determining when people should be released on bail. How do we ensure that those decisions are fair and reflect our societal values? This is an increasingly active area of research in the field.
As a person of color in machine learning, what are your thoughts on bringing more young people of color into this field?
There have been some developments in this direction that I’m particularly excited about. There’s a “Black in AI” movement now. It’s bringing people of African ancestry together in this field. Although we might be sparse and distributed, we have a big presence. It’s been excellent for networking and for encouraging younger people to get involved in machine learning and stay involved. You can learn more about it at blackinai.github.io or by searching online for Black in AI.
What books are you reading now? And what books inspired you when you were younger?
I just finished reading Astrophysics for People in a Hurry by Neil deGrasse Tyson, and now I’m in the middle of American Nations by Colin Woodard.
When I was younger, my three favorite books were Brian Greene’s The Fabric of the Cosmos, Matt Ridley’s The Red Queen and Neil Gaiman’s American Gods.
The world faces so many challenges today. What keeps you up at night?
I would say poverty. I can’t comprehend how there can be so much wealth in my field, my community and this country, and yet half a million people in the United States are homeless on any given night. One in nine people are malnourished in the world. That just doesn’t make sense to me.