One eighth grader is using AI to improve 911 response times
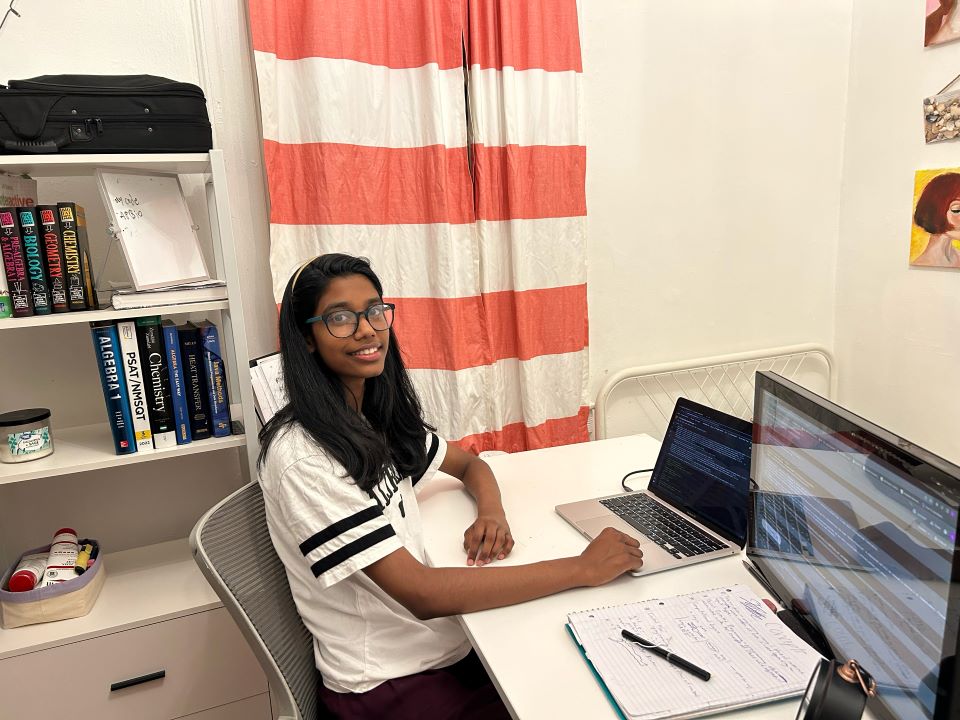
For the fifth year, The Lemelson Foundation is giving $100 awards to outstanding young inventors in Society Affiliate Fairs with middle school participants around the country. The prize was created to reward young inventors whose projects exemplify the ideals of inventive thinking by identifying challenges in their communities and creating solutions that will improve lives.
When an emergency takes place, we all know to call 911. Unfortunately, sometimes the system can break down with horrible consequences. In the winter of 2022, Lehansa Marambage, a student at Sara M. Gilmore Academy, witnessed a terrible tragedy: her 25 year old upstairs neighbor died after experiencing a seizure. Lehansa’s father called 911 but it took more than 40 minutes for help to arrive. By that time, it was too late.
“After researching, I pinpointed the delays to the newly regionalized call systems employed as cost- saving measures in my city,” Lehansa explained. “Human error is inevitable, but at what cost? I wanted answers.”
At the time, Lehansa was taking a Python programming class at the New Jersey Institute of Technology and a machine learning bootcamp called Inspirit AI, offered by MIT and Stanford. “The idea of creating a machine learning model came to my mind. I wanted to use technology to help minimize human errors and prevent death by saving time,” explained Lehansa.
This inquiry led Lehansa, then an 8th grader, to develop an “AI Department Prediction System” that she created and trained using a 911 distress call dataset and a machine learning model. In short, her project used artificial intelligence (AI) to remake aspects of the 911 computer aided dispatch (CAD) for efficiency, in terms of speed and accuracy. For her hypothesis, Lehansa believed that if natural language programming (NLP) and AI were used to develop the 911 call and dispatch system, it would enable a faster, more effective response to emergencies.
The AI Department Prediction System first passes audio clips of distress calls into the machine-learning model—into the input layer. After getting the input data, the model pushes the clip through multiple hidden layers of a deep learning neural network. The output layer of the model results in a classification of the call/audio clip into an emergency category, such as fire, EMS or police. Depending on the classification, the program then dials the corresponding emergency department. The system would also transcribe the call/audio clip.
Lehansa shared that the seconds and minutes it takes for operators to pull up protocol-documents and ring up their local fire, police or EMS number can create a bottleneck for hundreds of other callers that are also experiencing emergencies. “With a few integrations, my program can help identify, understand and react to a variety of distress calls,” she said. “Overall, compared to older systems, the newly created 911 CAD system features were much more efficient in regard to the time and effort required.”
When considering her favorite part of the invention process and developing this system, Lehansa said that she enjoyed the brainstorming and design stage the most because “the sky is the limit in this phase.” The most difficult part was debugging her code, she noted.
Lehansa said she was honored to receive the Lemelson Early Inventor Prize at the Jersey City Medical Center/Barnabas Health STEM Showcase. “I am grateful to have been recognized in my attempt to better the world, as this is a chance for my invention to reach more people and hopefully help me further develop my project in order to save the lives of millions of Americans across the nation.”